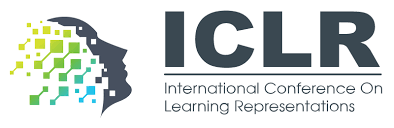
Two DSP group papers have been accepted as "Notable - Top 25%" papers for the International Conference on Learning Representations (ICLR) 2023 in Kigali, Rwanda
- "A Primal-Dual Framework for Transformers and Neural Networks," by T. M. Nguyen, T. Nguyen, N. Ho, A. L. Bertozzi, R. G. Baraniuk, and S. Osher
- "Retrieval-based Controllable Molecule Generation," by Jack Wang, W. Nie, Z. Qiao, C. Xiao, R. G. Baraniuk, and A. Anandkumar
Abstracts below.
Retrieval-based Controllable Molecule Generation
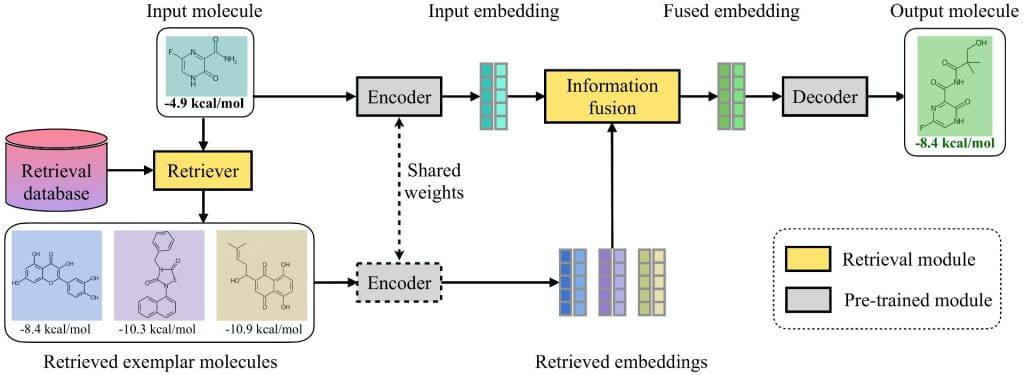
Generating new molecules with specified chemical and biological properties via generative models has emerged as a promising direction for drug discovery. However, existing methods require extensive training/fine-tuning with a large dataset, often unavailable in real-world generation tasks. In this work, we propose a new retrieval-based framework for controllable molecule generation. We use a small set of exemplar molecules, i.e., those that (partially) satisfy the design criteria, to steer the pre-trained generative model towards synthesizing molecules that satisfy the given design criteria. We design a retrieval mechanism that retrieves and fuses the exemplar molecules with the input molecule, which is trained by a new self-supervised objective that predicts the nearest neighbor of the input molecule. We also propose an iterative refinement process to dynamically update the generated molecules and retrieval database for better generalization. Our approach is agnostic to the choice of generative models and requires no task-specific fine-tuning. On various tasks ranging from simple design criteria to a challenging real-world scenario for designing lead compounds that bind to the SARS-CoV-2 main protease, we demonstrate our approach extrapolates well beyond the retrieval database, and achieves better performance and wider applicability than previous methods.
A Primal-Dual Framework for Transformers and Neural Networks
Self-attention is key to the remarkable success of transformers in sequence modeling tasks, including many applications in natural language processing and computer vision. Like neural network layers, these attention mechanisms are often developed by heuristics and experience. To provide a principled framework for constructing attention layers in transformers, we show that the self-attention corresponds to the support vector expansion derived from a support vector regression problem, whose primal formulation has the form of a neural network layer. Using our framework, we derive popular attention layers used in practice and propose two new attentions: 1) the Batch Normalized Attention (Attention-BN) derived from the batch normalization layer and 2) the Attention with Scaled Head (Attention-SH) derived from using less training data to fit the SVR model. We empirically demonstrate the advantages of the Attention-BN and Attention-SH in reducing head redundancy, increasing the model’s accuracy, and improving the model’s efficiency in a variety of practical applications including image and time-series classification.