"A Blessing of Dimensionality in Membership Inference through Regularization" by DSP group members Jasper Tan, Daniel LeJeune, Blake Mason, Hamid Javadi, and Richard Baraniuk has been accepted for the International Conference on Artificial Intelligence and Statistics (AISTATS) in Valencia, Spain, April 2023.
Author Archives: jkh6
Two “Notable” Papers at ICLR 2023
Two DSP group papers have been accepted as "Notable - Top 25%" papers for the International Conference on Learning Representations (ICLR) 2023 in Kigali, Rwanda
- "A Primal-Dual Framework for Transformers and Neural Networks," by T. M. Nguyen, T. Nguyen, N. Ho, A. L. Bertozzi, R. G. Baraniuk, and S. Osher
- "Retrieval-based Controllable Molecule Generation," by Jack Wang, W. Nie, Z. Qiao, C. Xiao, R. G. Baraniuk, and A. Anandkumar
Abstracts below.
Retrieval-based Controllable Molecule Generation
Generating new molecules with specified chemical and biological properties via generative models has emerged as a promising direction for drug discovery. However, existing methods require extensive training/fine-tuning with a large dataset, often unavailable in real-world generation tasks. In this work, we propose a new retrieval-based framework for controllable molecule generation. We use a small set of exemplar molecules, i.e., those that (partially) satisfy the design criteria, to steer the pre-trained generative model towards synthesizing molecules that satisfy the given design criteria. We design a retrieval mechanism that retrieves and fuses the exemplar molecules with the input molecule, which is trained by a new self-supervised objective that predicts the nearest neighbor of the input molecule. We also propose an iterative refinement process to dynamically update the generated molecules and retrieval database for better generalization. Our approach is agnostic to the choice of generative models and requires no task-specific fine-tuning. On various tasks ranging from simple design criteria to a challenging real-world scenario for designing lead compounds that bind to the SARS-CoV-2 main protease, we demonstrate our approach extrapolates well beyond the retrieval database, and achieves better performance and wider applicability than previous methods.
A Primal-Dual Framework for Transformers and Neural Networks
Self-attention is key to the remarkable success of transformers in sequence modeling tasks, including many applications in natural language processing and computer vision. Like neural network layers, these attention mechanisms are often developed by heuristics and experience. To provide a principled framework for constructing attention layers in transformers, we show that the self-attention corresponds to the support vector expansion derived from a support vector regression problem, whose primal formulation has the form of a neural network layer. Using our framework, we derive popular attention layers used in practice and propose two new attentions: 1) the Batch Normalized Attention (Attention-BN) derived from the batch normalization layer and 2) the Attention with Scaled Head (Attention-SH) derived from using less training data to fit the SVR model. We empirically demonstrate the advantages of the Attention-BN and Attention-SH in reducing head redundancy, increasing the model’s accuracy, and improving the model’s efficiency in a variety of practical applications including image and time-series classification.
OpenStax Research Website Now Live
Check out the new website for the awesome OpenStax Research team!
Jasper Tan Defends PhD Thesis
Rice DSP graduate student Jasper Tan successfully defended his PhD thesis entitled "Privacy-Preserving Machine Learning: The Role of Overparameterization and Solutions in Computational Imaging.”
Abstract: While the accelerating deployment of machine learning (ML) models brings benefits to various aspects of human life, it also opens the door to serious privacy risks. In particular, it is sometimes possible to reverse engineer a given model to extract information about the data on which it was trained. Such leakage is especially dangerous if the model's training data contains sensitive information, such as medical records, personal media, or consumer behavior. This thesis is concerned with two big questions around this privacy issue: (1) "what makes ML models vulnerable to privacy attacks?" and (2) "how do we preserve privacy in ML applications?". For question (1), I present detailed analysis on the effect increased overparameterization has on a model's vulnerability to the membership inference (MI) privacy attack, the task of identifying whether a given point is included in the model's training dataset or not. I theoretically and empirically show multiple settings wherein increased overparameterization leads to increased vulnerability to MI even while improving generalization performance. However, I then show that incorporating proper regularization while increasing overparameterization can eliminate this effect and can actually increase privacy while preserving generalization performance, yielding a "blessing of dimensionality’" for privacy through regularization. For question (2), I present results on the privacy-preserving techniques of synthetic training data simulation and privacy-preserving sensing, both in the domain of computational imaging. I first present a training data simulator for accurate ML-based depth of field (DoF) extension for time-of-flight (ToF) imagers, resulting in a 3.6x increase in a conventional ToF camera's DoF when used with a deblurring neural network. This simulator allows ML to be used without the need for potentially private real training data. Second, I propose a design for a sensor whose measurements obfuscate person identities while still allowing person detection to be performed. Ultimately, it is my hope that these findings and results take the community one step closer towards the responsible deployment of ML models without putting sensitive user data at risk.
Jasper’s next step is the start-up GLASS Imaging, where he will be leveraging machine learning and computational photography techniques to design a new type of camera with SLR image quality that can fit in your pocket.
DSP Faculty Member Richard Baraniuk Honored with IEEE SPS Norbert Wiener Society Award
Richard Baraniuk has been selected for the 2022 IEEE SPS Norbert Wiener Society Award "for fundamental contributions to sparsity-based signal processing and pioneering broad dissemination of open educational resources". The Society Award honors outstanding technical contributions in a field within the scope of the IEEE Signal Processing Society and outstanding leadership in that field (list of previous recipients).
Why Some Deep Networks are Easier to Optimize Than Others
"Singular Value Perturbation and Deep Network Optimization", Rudolf H. Riedi, Randall Balestriero, and Richard G. Baraniuk, Constructive Approximation, 27 November 2022 (also arXiv preprint 2203.03099, 7 March 2022)
Deep learning practitioners know that ResNets and DenseNets are much preferred over ConvNets, because empirically their gradient descent learning converges faster and more stably to a better solution. In other words, it is not what a deep network can approximate that matters, but rather how it learns to approximate. Empirical studies have indicated that this is because the so-called loss landscape of the objective function navigated by gradient descent as it optimizes the deep network parameters is much smoother for ResNets and DenseNets as compared to ConvNets (see Figure 1 from Tom Goldstein's group below). However, to date there has been no analytical work in this direction.
Building on our earlier work connecting deep networks with continuous piecewise-affine splines, we develop an exact local linear representation of a deep network layer for a family of modern deep networks that includes ConvNets at one end of a spectrum and networks with skip connections, such as ResNets and DenseNets, at the other. For tasks that optimize the squared-error loss, we prove that the optimization loss surface of a modern deep network is piecewise quadratic in the parameters, with local shape governed by the singular values of a matrix that is a function of the local linear representation. We develop new perturbation results for how the singular values of matrices of this sort behave as we add a fraction of the identity and multiply by certain diagonal matrices. A direct application of our perturbation results explains analytically why a network with skip connections (e.g., ResNet or DenseNet) is easier to optimize than a ConvNet: thanks to its more stable singular values and smaller condition number, the local loss surface of a network with skip connections is less erratic, less eccentric, and features local minima that are more accommodating to gradient-based optimization. Our results also shed new light on the impact of different nonlinear activation functions on a deep network's singular values, regardless of its architecture.
Daniel LeJeune Defends PhD Thesis
Rice DSP graduate student Daniel LeJeune successfully defended his PhD thesis entitled "Ridge Regularization by Randomization in Linear Ensembles".
Abstract: Ensemble methods that average over a collection of independent predictors that are each limited to random sampling of both the examples and features of the training data command a significant presence in machine learning, such as the ever-popular random forest. Combining many such randomized predictors into an ensemble produces a highly robust predictor with excellent generalization properties; however, understanding the specific nature of the effect of randomization on ensemble method behavior has received little theoretical attention.We study the case of an ensembles of linear predictors, where each individual predictor is a linear predictor fit on a randomized sample of the data matrix. We first show a straightforward argument that an ensemble of ordinary least squares predictors fit on a simple subsampling can achieve the optimal ridge regression risk in a standard Gaussian data setting. We then significantly generalize this result to eliminate essentially all assumptions on the data by considering ensembles of linear random projections or sketches of the data, and in doing so reveal an asymptotic first-order equivalence between linear regression on sketched data and ridge regression. By extending this analysis to a second-order characterization, we show how large ensembles converge to ridge regression under quadratic metrics.
Daniel's next step is a postdoc with Emmanuel Candes at Stanford University.
New Course for Spring 2023: ELEC378 – Machine Learning: Concepts and Techniques
ELEC378 - Machine Learning: Concepts and Techniques
Instructor: Prof. Richard Baraniuk
Machine learning is a powerful new way to build signal processing models and systems using data rather than physics. This introductory course covers the key ideas, algorithms, and implementations of both classical and modern methods. Topics include supervised and unsupervised learning, optimization, linear regression, logistic regression, support vector machines, deep neural networks, clustering, data mining. A course highlight is a hands-on team project competition using real-world data.
The course is open to students at all levels who are comfortable with linear algebra + coding in Python (ideally), R, MATLAB.
Course webpage; more information coming soon!
A Visual Tour of Current Challenges in Multimodal Language Models
A Visual Tour of Current Challenges in Multimodal Language Models
Shashank Sonkar, Naiming Liu, Richard G. Baraniuk
arXiv preprint 2210.12565
October 2022
Transformer models trained on massive text corpora have become the de facto models for a wide range of natural language processing tasks. However, learning effective word representations for function words remains challenging. Multimodal learning, which visually grounds transformer models in imagery, can overcome the challenges to some extent; however, there is still much work to be done. In this study, we explore the extent to which visual grounding facilitates the acquisition of function words using stable diffusion models that employ multimodal models for text-to-image generation. Out of seven categories of function words, along with numerous subcategories, we find that stable diffusion models effectively model only a small fraction of function words – a few pronoun subcategories and relatives. We hope that our findings will stimulate the development of new datasets and approaches that enable multimodal models to learn better representations of function words.
Above: Sample images depicting an SDM's success (green border) and failure (red border) in capturing the semantics of different subcategories of pronouns. (a)-(c) show that the information about gender and count implicit in subject pronouns like he, she, we is accurately depicted. But, for indefinite pronouns, SDMs fail to capture the notion of negatives ((d) nobody), existential ((e) some), and universals ((f) everyone). Likewise SDMs fail to capture the meaning of reflexive pronouns such as (g) myself, (h) himself, (i) herself.
We provide the code on github for readers to replicate our findings and explore further.
DSP PhD Student Jack Wang a University of Chicago “Rising Star in Data Science”
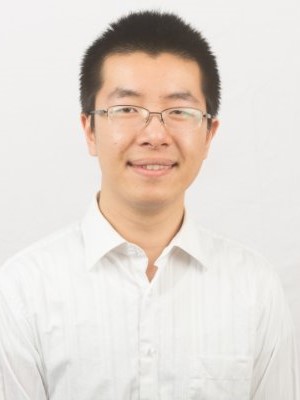